How to let a chatbot use a model already trained
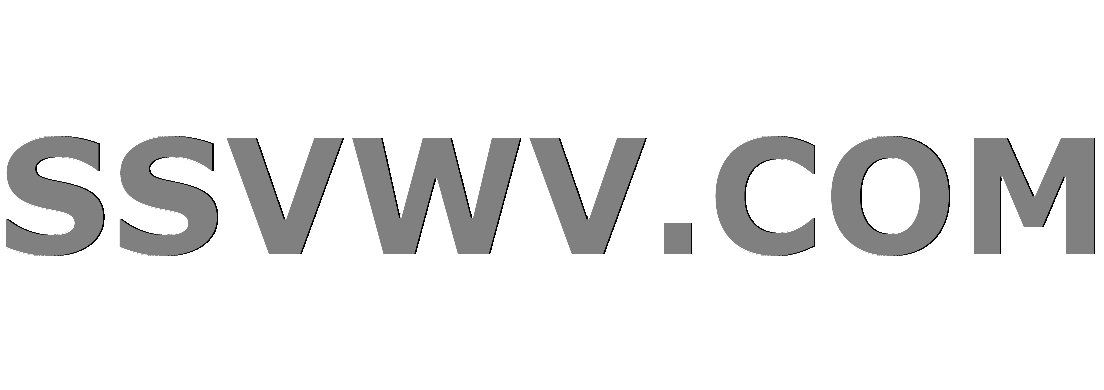
Multi tool use
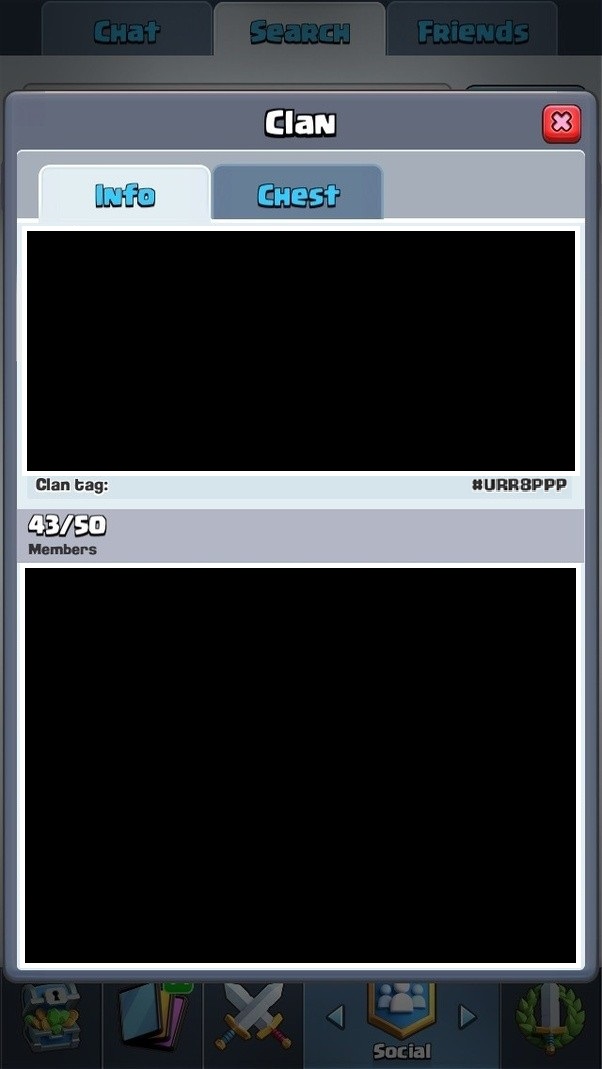

How to let a chatbot use a model already trained
I am trying for the first time to build a chatbot.
Following this tutorial, I already have a jupyter notebook solution that is working with a model already trained.
import nltk
from nltk.stem.lancaster import LancasterStemmer
import numpy as np
import tflearn
import tensorflow as tf
import random
import json
from ._conv import register_converters as _register_converters
stemmer = LancasterStemmer()
with open('intents.json') as json_data:
intents = json.load(json_data)
words =
classes =
documents =
ignore_words = ['?']
# loop through each sentence in our intents patterns
for intent in intents['intents']:
for pattern in intent['patterns']:
# tokenize each word in the sentence
w = nltk.word_tokenize(pattern)
# add to our words list
words.extend(w)
# add to documents in our corpus
documents.append((w, intent['tag']))
# add to our classes list
if intent['tag'] not in classes:
classes.append(intent['tag'])
# stem and lower each word and remove duplicates
words = [stemmer.stem(w.lower()) for w in words if w not in ignore_words]
words = sorted(list(set(words)))
# remove duplicates
classes = sorted(list(set(classes)))
print (len(documents), "documents")
print (len(classes), "classes", classes)
print (len(words), "unique stemmed words", words)
20 documents
6 classes ['goodbye', 'greeting', 'hours', 'opentoday', 'payments', 'thanks']
32 unique stemmed words ["'s", 'acceiv', 'anyon', 'ar', 'bye', 'card', 'cash', 'credit', 'day', 'do', 'good', 'goodby', 'hello', 'help', 'hi', 'hour', 'how', 'is', 'lat', 'mastercard', 'on', 'op', 'see', 'tak', 'thank', 'that', 'ther', 'today', 'what', 'when', 'yo', 'you']
# create our training data
training =
output =
# create an empty array for our output
output_empty = [0] * len(classes)
# training set, bag of words for each sentence
for doc in documents:
# initialize our bag of words
bag =
# list of tokenized words for the pattern
pattern_words = doc[0]
# stem each word
pattern_words = [stemmer.stem(word.lower()) for word in pattern_words]
# create our bag of words array
for w in words:
bag.append(1) if w in pattern_words else bag.append(0)
# output is a '0' for each tag and '1' for current tag
output_row = list(output_empty)
output_row[classes.index(doc[1])] = 1
training.append([bag, output_row])
# shuffle our features and turn into np.array
random.shuffle(training)
training = np.array(training)
# create train and test lists
train_x = list(training[:,0])
train_y = list(training[:,1])
# reset underlying graph data
tf.reset_default_graph()
# Build neural network
net = tflearn.input_data(shape=[None, len(train_x[0])])
net = tflearn.fully_connected(net, 8)
net = tflearn.fully_connected(net, 8)
net = tflearn.fully_connected(net, len(train_y[0]), activation='softmax')
net = tflearn.regression(net)
# Define model and setup tensorboard
model = tflearn.DNN(net, tensorboard_dir='tflearn_logs')
# Start training (apply gradient descent algorithm)
model.fit(train_x, train_y, n_epoch=1000, batch_size=8, show_metric=True)
model.save('model.tflearn')
Training Step: 2999 | total loss: 0.01771 | time: 0.006s
| Adam | epoch: 1000 | loss: 0.01771 - acc: 0.9999 -- iter: 16/20
Training Step: 3000 | total loss: 0.01754 | time: 0.009s
| Adam | epoch: 1000 | loss: 0.01754 - acc: 1.0000 -- iter: 20/20
--
INFO:tensorflow:/home/marco/PycharmProjects/chatBot/model.tflearn is not in all_model_checkpoint_paths. Manually adding it.
# save all of our data structures
import pickle
pickle.dump( {'words':words, 'classes':classes, 'train_x':train_x, 'train_y':train_y}, open( "training_data", "wb" ) )
data = pickle.load( open( "training_data", "rb" ) )
words = data['words']
classes = data['classes']
train_x = data['train_x']
train_y = data['train_y']
# import our chat-bot intents file
import json
with open('intents.json') as json_data:
intents = json.load(json_data)
# load our saved model
model.load('./model.tflearn')
INFO:tensorflow:Restoring parameters from /home/marco/PycharmProjects/chatBot/model.tflearn
def clean_up_sentence(sentence):
# tokenize the pattern
sentence_words = nltk.word_tokenize(sentence)
# stem each word
sentence_words = [stemmer.stem(word.lower()) for word in sentence_words]
return sentence_words
# return bag of words array: 0 or 1 for each word in the bag that exists in the sentence
def bow(sentence, words, show_details=False):
# tokenize the pattern
sentence_words = clean_up_sentence(sentence)
# bag of words
bag = [0]*len(words)
for s in sentence_words:
for i,w in enumerate(words):
if w == s:
bag[i] = 1
if show_details:
print ("found in bag: %s" % w)
return(np.array(bag))
# create a data structure to hold user context
context = {}
ERROR_THRESHOLD = 0.25
def classify(sentence):
# generate probabilities from the model
results = model.predict([bow(sentence, words)])[0]
# filter out predictions below a threshold
results = [[i,r] for i,r in enumerate(results) if r>ERROR_THRESHOLD]
# sort by strength of probability
results.sort(key=lambda x: x[1], reverse=True)
return_list =
for r in results:
return_list.append((classes[r[0]], r[1]))
# return tuple of intent and probability
return return_list
def response(sentence, userID='123', show_details=False):
results = classify(sentence)
# if we have a classification then find the matching intent tag
if results:
# loop as long as there are matches to process
while results:
for i in intents['intents']:
# find a tag matching the first result
if i['tag'] == results[0][0]:
# set context for this intent if necessary
if 'context_set' in i:
if show_details: print ('context:', i['context_set'])
context[userID] = i['context_set']
# check if this intent is contextual and applies to this user's conversation
if not 'context_filter' in i or
(userID in context and 'context_filter' in i and i['context_filter'] == context[userID]):
if show_details: print ('tag:', i['tag'])
# a random response from the intent
return print(random.choice(i['responses']))
results.pop(0)
Example of output:
response('Hello')
>>Hi there, how can I help?
response('open')
>>Our hours are 9am-8pm every day
My goal is to structure the chatbot itself using object oriented programming (with a base structure I thought about below):
class Bot:
'''This class defines the routine of the bot'''
def __init__(self, presentation):
'''The bot presents itself'''
self.presentation = presentation
print('')
print(presentation)
def ask_question(self):
'''This method defines how the bot asks questions'''
print('')
self.answer = str(input('Please enter your question: ')).split(' ')
print('')
print('Thank you for your question. Let me check..')
def answer_question(self):
'''This method answer the user's questions'''
print('')
print(self.answer)
bot = Bot('Good morning, my Name is BotPy')
question = bot.ask_question()
answer = bot.answer_question()
My question is: Do I have to train the model every time I launch the chatbot, or I can call the model already trained from the directory? If yes, how?
By clicking "Post Your Answer", you acknowledge that you have read our updated terms of service, privacy policy and cookie policy, and that your continued use of the website is subject to these policies.