Finetuning ResNet50 with keras - val_loss keeps increasing
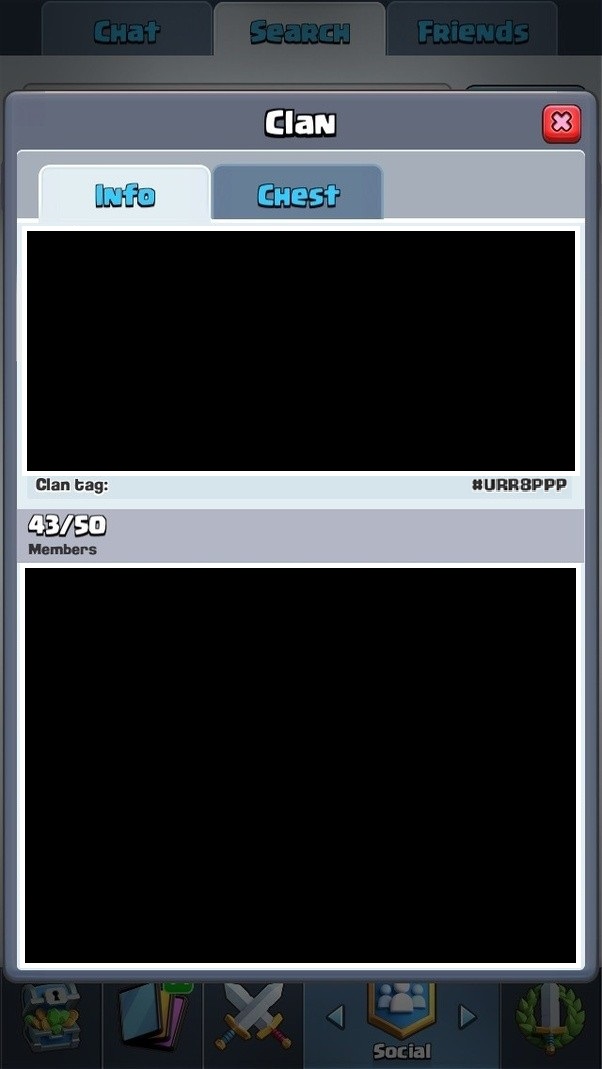

Finetuning ResNet50 with keras - val_loss keeps increasing
I am trying to customize resnet50 using keras with a tensorflow backend. However, upon tranining my val_loss keeps increasing. Trying different learning rates and batch sizes does not resolve the problem.
Using different preprocessing methods such as rescaling or using the preprocess_input function for resnet50 inside the ImageDataGenerator did not not solve the problem either.
This is the code I am using
Importing and preprocessing data:
from keras.preprocessing.image import ImageDataGenerator
from keras.applications.resnet50 import preprocess_input, decode_predictions
IMAGE_SIZE = 224
BATCH_SIZE = 32
num_classes = 27
main_path = "C:/Users/aaron/Desktop/DATEN/data"
gesamt_path = os.path.join(main_path, "ML_DATA")
labels = listdir(gesamt_path)
data_generator = ImageDataGenerator(#rescale=1./255,
validation_split=0.20,
preprocessing_function=preprocess_input)
train_generator = data_generator.flow_from_directory(gesamt_path, target_size=(IMAGE_SIZE, IMAGE_SIZE), shuffle=True, seed=13,
class_mode='categorical', batch_size=BATCH_SIZE, subset="training")
validation_generator = data_generator.flow_from_directory(gesamt_path, target_size=(IMAGE_SIZE, IMAGE_SIZE), shuffle=False, seed=13,
class_mode='categorical', batch_size=BATCH_SIZE, subset="validation")
Defining and training the model
img_width = 224
img_height = 224
model = keras.applications.resnet50.ResNet50()
classes = list(iter(train_generator.class_indices))
model.layers.pop()
for layer in model.layers:
layer.trainable=False
last = model.layers[-1].output
x = Dense(len(classes), activation="softmax")(last)
finetuned_model = Model(model.input, x)
finetuned_model.compile(optimizer=Adam(lr=0.001), loss='categorical_crossentropy', metrics=['accuracy'])
for c in train_generator.class_indices:
classes[train_generator.class_indices[c]] = c
finetuned_model.classes = classes
earlystopCallback = keras.callbacks.EarlyStopping(monitor='val_loss', min_delta=0, patience=8, verbose=1, mode='auto')
tbCallBack = keras.callbacks.TensorBoard(log_dir='./Graph', histogram_freq=0, write_graph=True, write_images=True)
history = finetuned_model.fit_generator(train_generator,
validation_data=validation_generator,
epochs=85, verbose=1,callbacks=[tbCallBack,earlystopCallback])
By clicking "Post Your Answer", you acknowledge that you have read our updated terms of service, privacy policy and cookie policy, and that your continued use of the website is subject to these policies.